일 | 월 | 화 | 수 | 목 | 금 | 토 |
---|---|---|---|---|---|---|
1 | 2 | 3 | 4 | |||
5 | 6 | 7 | 8 | 9 | 10 | 11 |
12 | 13 | 14 | 15 | 16 | 17 | 18 |
19 | 20 | 21 | 22 | 23 | 24 | 25 |
26 | 27 | 28 | 29 | 30 | 31 |
- 스몰토크
- 영어회화
- 링글경험담
- 둔촌역장어
- 장어랑고기같이
- 링글커리어
- #nlp
- CommunicateWiththeWorld
- 강동구장어맛집
- #링글
- 영어공부
- 해외취업컨퍼런스
- 영어공부법
- 뉴노멀챌린지
- #링글후기
- 화상영어
- #영어공부
- #체험수업
- 성내동장어
- 링글
- #Ringle
- 총각네장어
- 영어로전세계와소통하기
- 링글리뷰
- #영어발음교정
- 소통챌린지
- #직장인영어
- Ringle
- 오피스밋업
- 영어시험
- Today
- Total
목록Review of Papers (6)
Soohyun’s Machine-learning
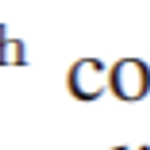
Contribution Suggest a way for improvement word-level language model with character level embeddings Pros and Cons of the Approach Used network in the paper was general things at the time, but input was different, character-level embeddings. The results was better if the language had more various morphemes. Yet character-level embeddings has a tradeoff between efficiency and time. Model Architec..
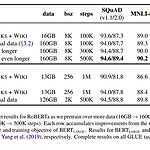
BERT가 충분하게 트레이닝되지 않았다-고 주장하고 시작한다. RoBERTa의 contribution 1) 더 나은 downstream task 성능을 낼 수 있는, BERT의 디자인 선택 (design choices), 그리고 트레이닝 전략 (training strategies)을 제시 2) CC-NEWS라는 새로운 데이터셋을 사용, 또한 사전학습(pretraining)에서 더 많은 데이터를 사용하는 것이 downstream tasks에서의 성능을 향상시키는 걸 확인 3) 트레이닝 향상은 masked language modeling이 올바르게 디자인 된 조건하에서, 최근에 발표된 방법들에 비견할만 함 RoBERTa (로베르타)의 특징 == 오리지널 BERT와의 차이점 1) dymanic masking ..
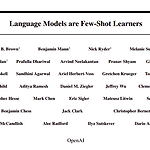
- GPT2의 계승 모델로, GPT3라고 부른다 - GPT는 Generative Pre-Training의 약자 (GPT1 논문 제목이 Improving Language Understanding by Generative Pre-Training) - input : N개의 단어 sequence - output : N+1번째의 단어 - GPT2 사이즈 업 + Unsupervised pre-training (like NLG) + Sparse Attention + No fine-tuning Alternating dense and Locally banded sparse attention - (a) Transaformer처럼 앞쪽의 전부를 보면 연산량이 많으므로, (b)나 (c)처럼 제한된 개수의 input token..
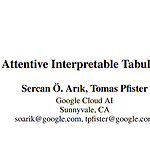
TabNet 라이브러리 깃허브 링크 : https://github.com/dreamquark-ai/tabnet Abstract TabNet uses sequential attention to choose which features to reason from at each decision step, enabling interpretability and more efficient learning as the learning capacity is used for the most salient features. keywords - interpretability - self-supervised learning - single deep learning architecture (for feature selection..
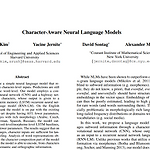
Contributioncharacter level의 embedding을 통해 word-level language model의 성능을 향상하는 방법을 제시했다.(네트워크 자체는 당시 보편적인 걸 사용했지만, input으로 char-level을 사용함으로써 word-level embedding이 정말 필요한 것인가-라는 의문을 제기한다. 영어, 체코어, 독일어, 스페인어, 불어, 러시아어, 아랍어 데이터셋으로 실험을 진행했다. 형태소가 풍부한 언어일수록 성능차이가 word-level 대비 더 좋게 나왔다. 여기까지가 장점이며 단점으로는 성능은 괜찮지만 char-level 자체가 efficiency - time tradeoff가 있다.) Abstract 우리는 오로지 Char-level inputs에만 의..
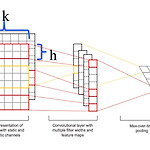
Novelty 1) very fast and strong with a single CNN layer (이전에도 CNN 쓴 논문들은 있었으나, 큰 효과를 보지는 못함) 2) pre-trained word vector 사용 (google negative300.bin download link) a summary of Abstract & Model architecture We report on a series of experiments with CNN trained on top of pre-trained word vectors for sentence-level classification tasks. We show that a simple CNN with little hyperparameter tuning and..